The Future of AI: From Unconscious Models to Conscious Reasoning and Emotional Intelligence
(May 26, 11:00–12:00)
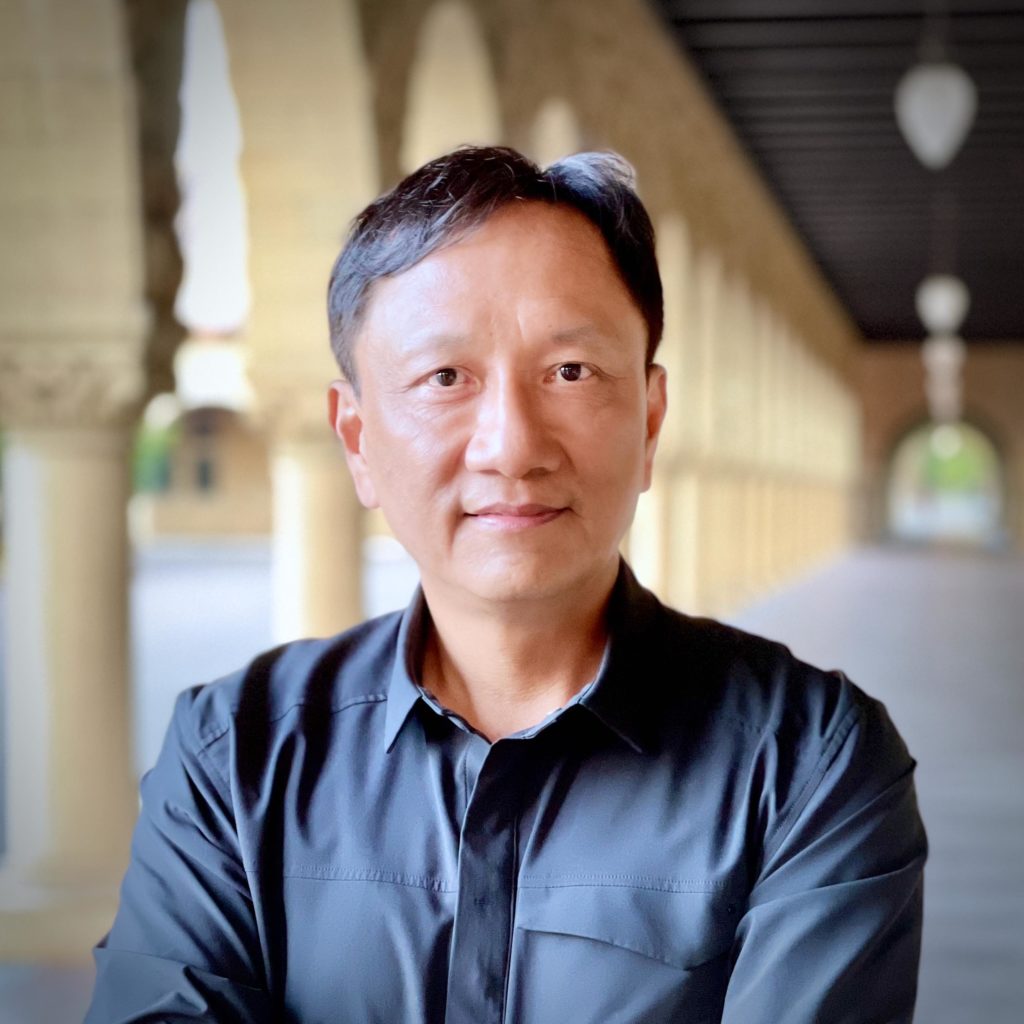
Dr. Edward Y. Chang
Founder/CTO, Ailly Corp.
Adjunct Professor, Computer Science, Stanford University
Abstract:
In this presentation, I first highlight the advancements in foundation models in the past decade, and present their impact on various domains. I then explore some known issues such as a lack of robustness, interpretability, and generalization. It’s argued that AI models have successfully modeled human unconsciousness to a certain extent. However, to enable AI to think and plan, a proposition is made [1] to model consciousness on top of unconsciousness. The discussion begins with the definition of consciousness, the exploration of the transitional mechanisms between consciousness and unconsciousness, theories of consciousness, and the differentiation between attention and consciousness. A functionalist approach is adopted to formulate a model of consciousness that encompasses perception, awareness, attention, critical thinking, creative thinking, and emotional intelligence. An illustration is provided on how these consciousness capabilities can be developed in foundation models through the careful design of prompt templates. Specifically, how the Socratic method [2], along with inductive, deductive, and abductive reasoning, can facilitate critical thinking and reading via prompt-template engineering is demonstrated. The integration of emotional intelligence to guide exploratory thinking, with ethical safeguards that respect individual and cultural values, is also discussed.
- CoCoMo: Computational Consciousness Modeling for Generative and Ethical AI, Edward Y. Chang, ArXiv:2304.02438 e-print, February, 2023. PDF
- Prompting Large Language Models With the Socratic Method, Edward Y. Chang, IEEE 13th Annual Computing and Communication Workshop and Conference (CCWC), March 2023. PDF
Biography:
Edward Y. Chang is an adjunct professor of Computer Science at Stanford University since 2019, and a visiting chair professor at Asia University. His current research interests include consciousness modeling, generative AI, and healthcare. Chang received his MS in CS and PhD in EE, both from Stanford University. He joined the ECE department of UC Santa Barbara in 1999, where he was tenured in 2003 and promoted to full professor in 2006. From 2006 to 2012, Chang served at Google as a director of research, leading research and development in areas such as scalable machine learning, indoor localization, Google QA, and recommendation systems. In subsequent years, Chang served as the president of HTC Healthcare (2012-2021) and a visiting professor at UC Berkeley AR/VR center (2017-2021), working on healthcare projects including VR surgery planning, AI-powered medical IoTs, and disease diagnosis. Between 2019 and 2022, Chang also served at SmartNews, a Tokyo-based unicorn, as its chief NLP advisor. Chang is an ACM fellow and IEEE fellow for his contributions to scalable machine learning and healthcare.
Unlocking the Power of Knowledge Graphs through Advanced Representation Learning
(May 27, 9:30–10:30)
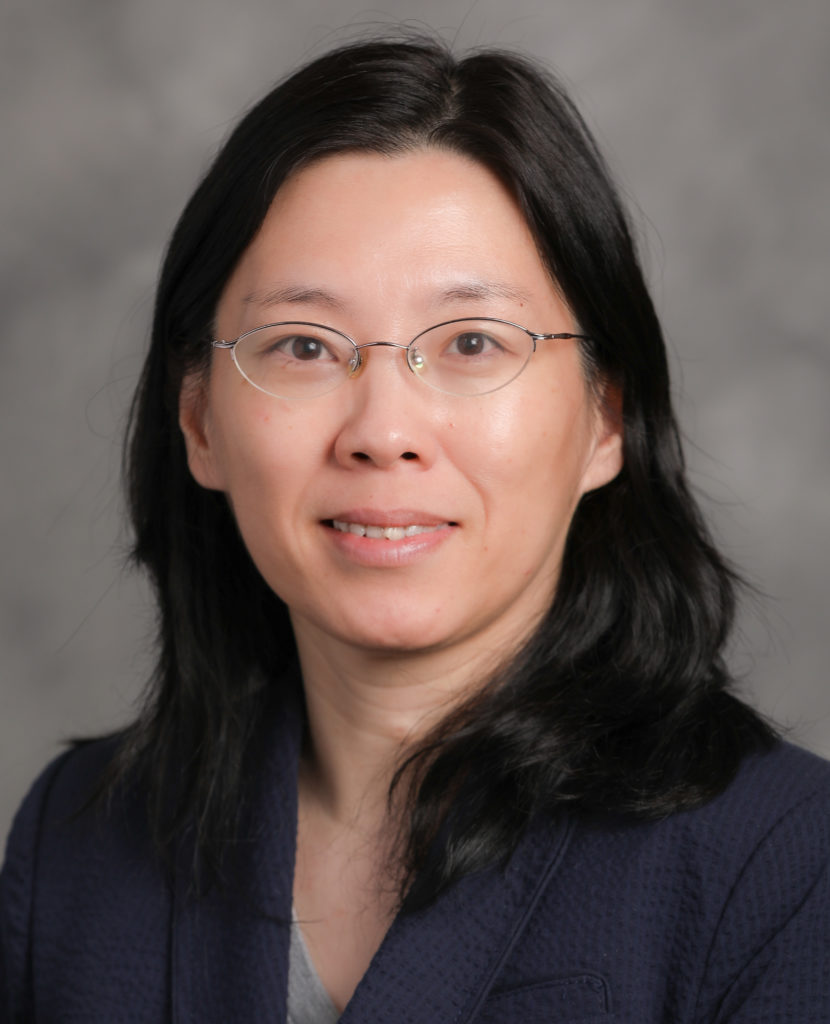
Dr. Wei Wang
Leonard Kleinrock Chair Professor, Department of Computer Science, University of California
Abstract:
Knowledge graphs are a powerful tool for representing complex, structured information about entities and their relationships. However, to fully unlock the potential of knowledge graphs, it is crucial to develop advanced representation learning techniques that can capture the complex semantics of entities and relationships. In this keynote speech, we will explore the latest advancements in knowledge graph representation learning, including our recent work on knowledge graph representation learning in non-Euclidean space. Non-Euclidean spaces provide more flexible and expressive representations of entities and relationships that can better capture the underlying semantics and structure of a knowledge graph. We will discuss various approaches to knowledge graph representation learning in non-Euclidean space, including hyperbolic space embeddings, spherical space embeddings, and other non-Euclidean spaces. We will also explore the potential of training multi-view embeddings that combine the advantages of multiple geometric spaces. These multi-view embeddings can provide a richer representation of entities and relationships, capturing complementary aspects of the underlying semantics and structure of a knowledge graph. We will highlight the challenges and opportunities in advancing knowledge graph representation learning, including scalability, interpretability, and multi-modal representation learning. Finally, we will discuss applications of knowledge graph representation learning in non-Euclidean space, such as knowledge graph completion, link prediction, and entity classification, among others.
Biography:
Wei Wang is the Leonard Kleinrock Chair Professor in Computer Science and Computational Medicine at University of California, Los Angeles and the director of the Scalable Analytics Institute (ScAi). She has extensive expertise in artificial intelligence, big data analytics, data mining, machine learning, natural language processing, bioinformatics and computational biology, and computational medicine. Her contributions to the field are significant, including seven filed patents, one monograph, and over 300 research papers in international journals and major peer-reviewed conference proceedings, with multiple best paper awards. Dr. Wang has been recognized with numerous awards and honors, including the IBM Invention Achievement Awards, an NSF Faculty Early Career Development (CAREER) Award, a Microsoft Research New Faculty Fellow Award, a Ruth Hettleman Prize for Artistic and Scholarly Achievement, an IEEE ICDM Outstanding Service Award, an Okawa Foundation Research Award, and an ACM SIGKDD Service Award. She has served as an associate editor of several prestigious academic journals and has held leadership positions in various academic conferences, including KDD, ICDM, and SDM, among others. She is the Chair of the ACM Special Interest Group on Knowledge Discovery in Data (SIGKDD). Dr. Wang is a fellow of both ACM and IEEE, demonstrating her outstanding contributions to the field.
Measurement Informatics: Interdisciplinary innovation of measurement and information science
(May 28, 9:30–10:30)
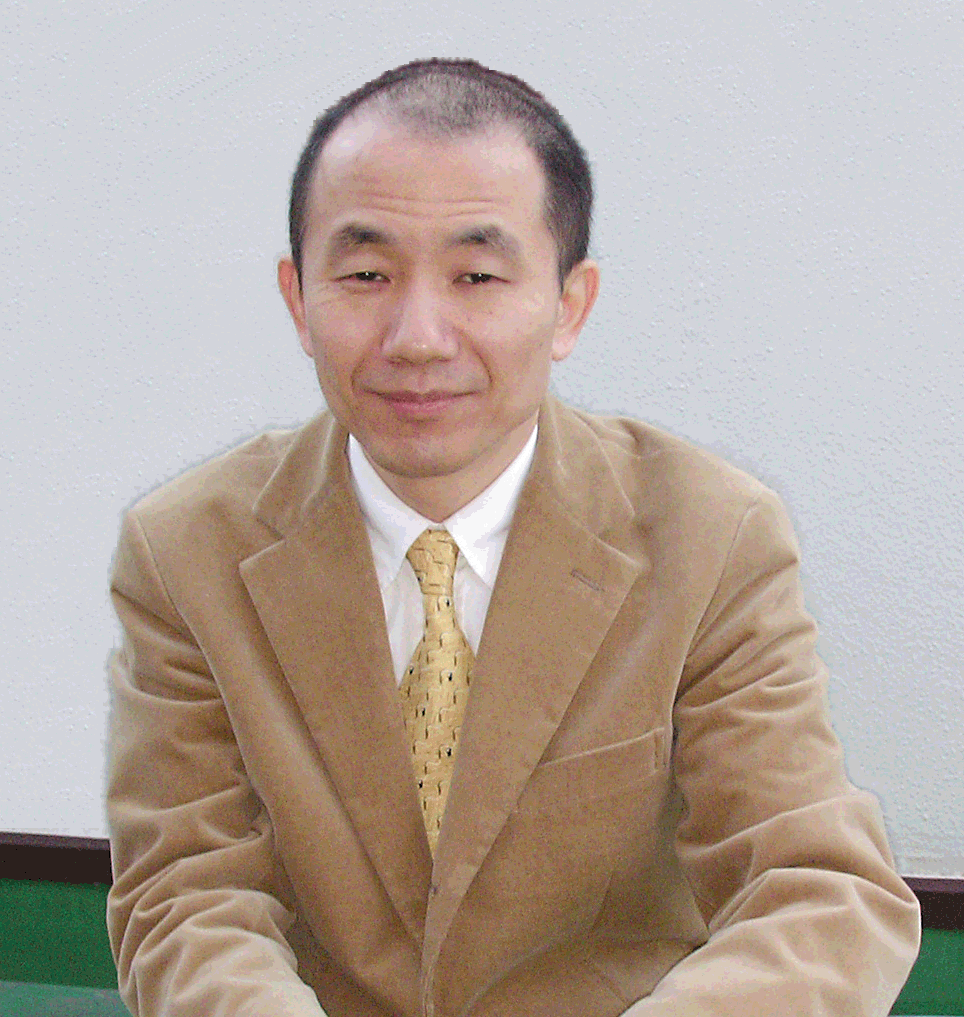
Dr. Takashi Washio
Professor, The Institute of Scientific and Industrial Research (ISIR: SANKEN), Osaka University
Abstract:
We are now entering an era where artificial intelligence and data mining are practically implemented in various domains, and scientific measurement and IoT sensing are such promising fields. Measurement Informatics is an interdisciplinary research of machine learning and statistical analysis based on systematical integration of the two sciences. Its outcomes are utilized in the development of advanced measurement devices to achieve superior measurement performance. Moreover, insights and experiences obtained in the research give us chances to create novel principles of information science. In this talk, important research issues and some approaches in Measurement Informatics are presented, and several concrete research examples such as ultra-high-speed super-resolution imaging of cell fluorescence and super-robust artificial olfactory sensing are demonstrated.
Biography:
Takashi Washio is a full professor at the Institute of Scientific and Industrial Research, Osaka University, a cross appointment director of AI Cooperative Research Laboratory of NEC Corporation and National Institute of Advanced Industrial Science and Technology and a director of KOBELCO Future Pioneering Co-Creation Research Center, Osaka University. His research group works on cutting-edge studies on machine learning and data mining. His recent interest is to develop novel machine/deep learning methods for measurements using advanced sensing devices. He has published more than 280 papers in major international conferences and journals including NIPS, ICML, AISTATS, SIG-KDD, ICDM, SDM, IJCAI, AAAI, JMLR, MLJ, TKDD, TKDE, DMKD, KAIS and AIJ. He is currently an associate editor/editorial board member of journals including TKDD, TKDE, DMKD and KAIS. He served the general co-chair of ICDM2022 and PC chairs of many major conferences.